Back to Applications
Agriculture
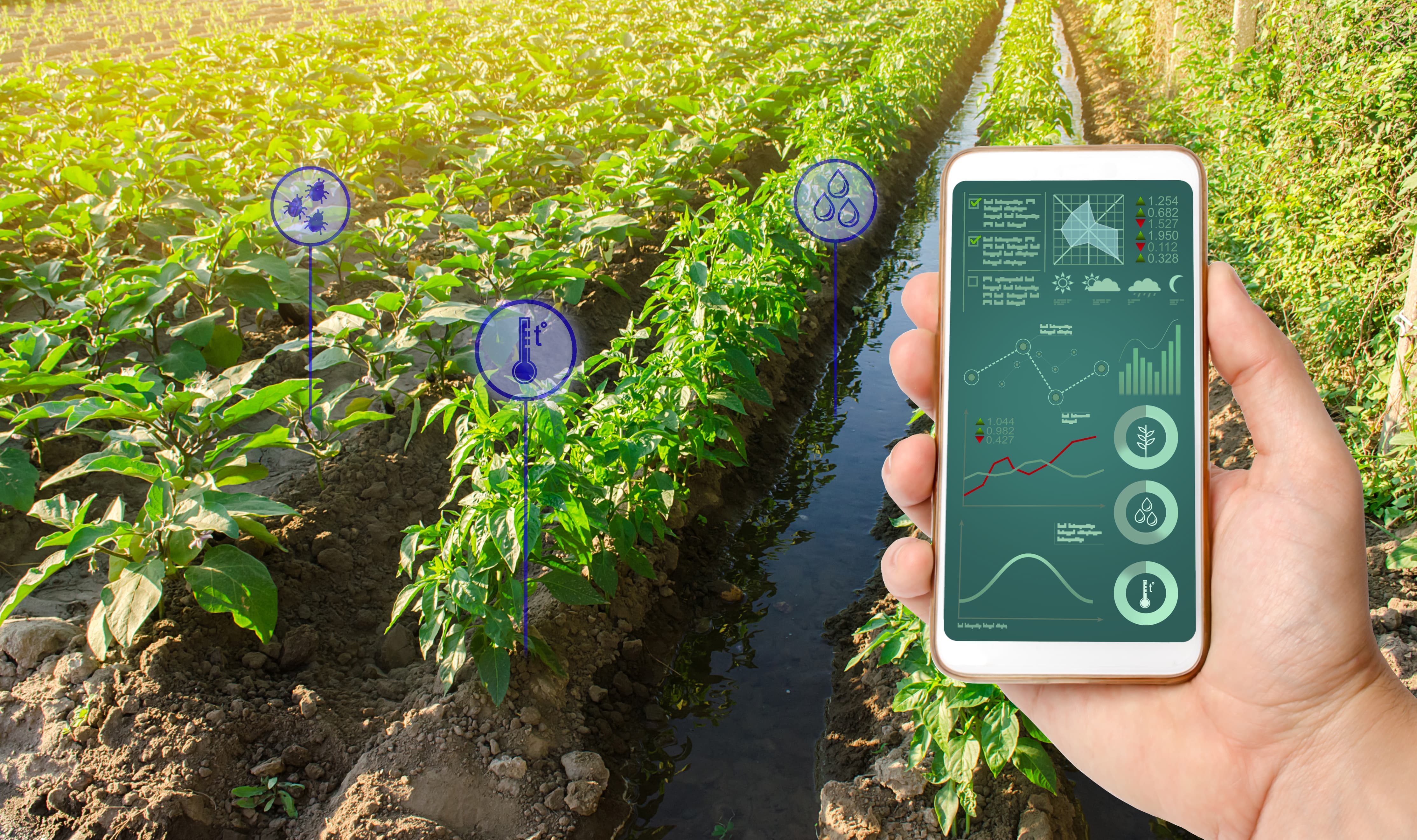
Crop Monitoring
Machine vision is critical to the farming revolution that is occurring right now. In the past, farmers often had to water or treat entire crops with pesticides because it was impossible to keep track of specific areas. But machine vision-enabled cameras and drones can keep a constant “eye” on the field. Always-on surveillance systems alert farmers when they need to remedy disease or drought.
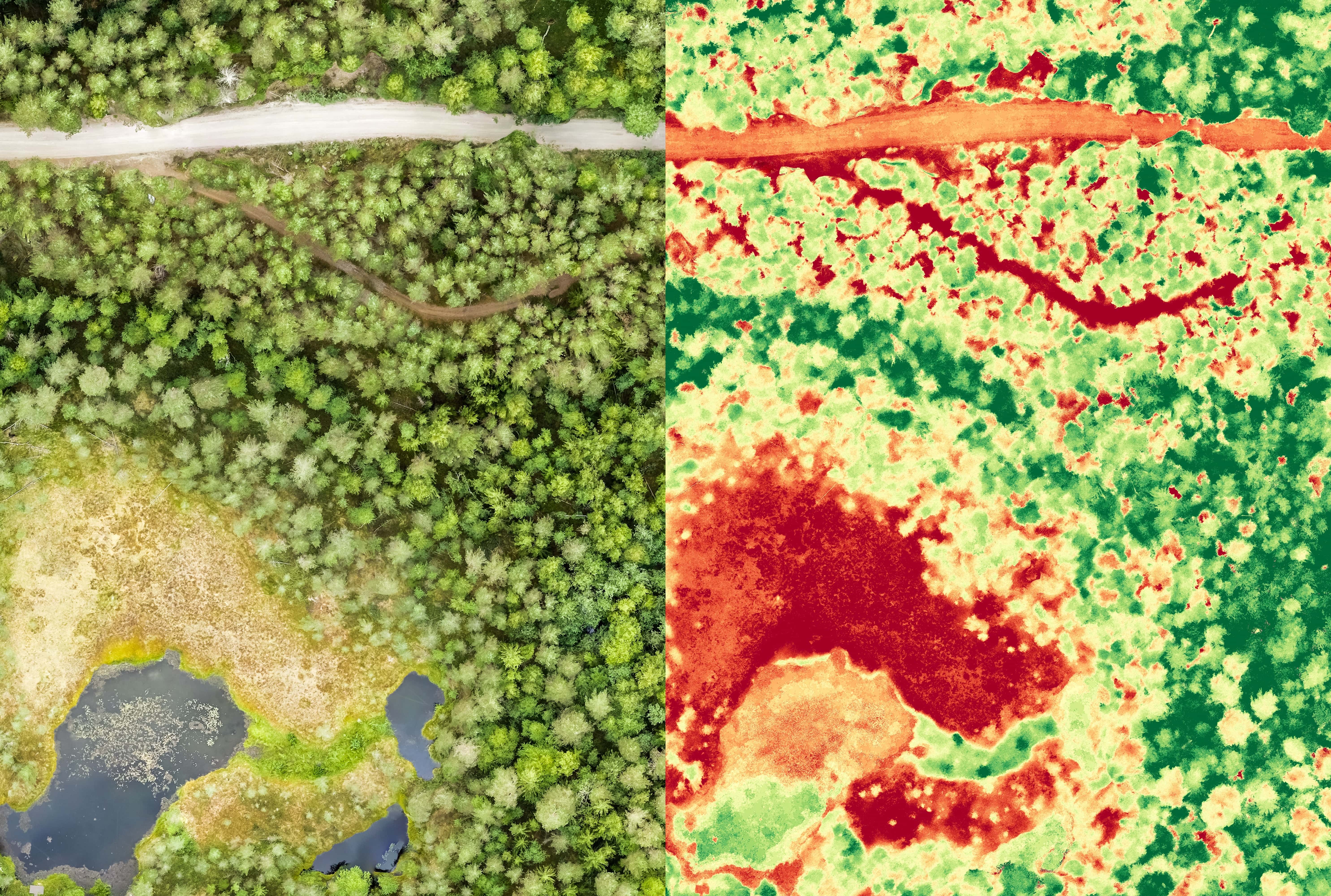
Pest & Disease Detection
Machine vision cameras are used in pest and disease detection in agriculture, offering a non-invasive and efficient method for monitoring crop health and identifying potential threats. These cameras, equipped with specialized sensors and imaging algorithms, capture high-resolution images of crops in fields or greenhouses. By analyzing the color, texture, and morphology of plant leaves and fruits, machine vision cameras can detect subtle signs of pest infestation, disease symptoms, or nutrient deficiencies. Additionally, thermal imaging capabilities allow these cameras to identify temperature variations associated with stress or pathogen activity. By providing real-time monitoring and early detection of pests and diseases, machine vision cameras enable farmers to implement targeted interventions, such as precision spraying or localized treatments, reducing the need for broad-spectrum pesticides and minimizing crop losses. Moreover, with the integration of artificial intelligence algorithms, these cameras can continuously learn and improve their detection capabilities, enhancing accuracy and efficiency in pest and disease management strategies. Overall, machine vision cameras contribute to sustainable agriculture practices by enabling proactive and precise crop monitoring, ultimately supporting higher yields and healthier crops.
-min.jpeg)
Optical Sorting
Machine vision cameras are used in optical sorting systems for agriculture, facilitating the rapid and accurate identification and separation of agricultural products based on various quality parameters such as size, color, shape, and defect detection. These cameras, equipped with high-resolution sensors and advanced imaging algorithms, capture detailed images of fruits, vegetables, grains, or other agricultural products as they pass through conveyor belts or sorting platforms. By analyzing the visual characteristics of each item in real-time, machine vision cameras can classify them according to predefined criteria, such as ripeness, size uniformity, or the presence of defects. This information is then used to activate pneumatic jets or mechanical arms that divert the products into different bins or conveyors based on their quality attributes. By automating the sorting process, machine vision cameras improve efficiency, reduce labor costs, and ensure consistent product quality in agricultural processing facilities. Moreover, with the integration of artificial intelligence and machine learning techniques, these cameras can adapt to variations in product appearance or environmental conditions, further enhancing sorting accuracy and flexibility in addressing diverse market demands. Overall, machine vision cameras play a crucial role in optimizing agricultural production and supply chain management by enabling precise and efficient optical sorting operations.
.jpeg)
Package Inspection
When it comes to sorting letters and boxes, machine vision handles the three most common types: post (or letter); parcel, usually measuring up to 40 inches on a side; and carton, which contain multiple parcels, products, etc. These three applications can be further characterized as 2D and 3D applications. Letters, envelopes and other “postal” products lay flat on conveyors. Postal applications may require the vision system to measure the size of the envelop to confirm a shipping rate, but mainly these applications use Optical Character Recognition (OCR) to read either hand written or machine written addresses, as well as some barcode or data matrix reading for bulk mail. This information is then used to automatically sort the mail according to its destination. Color is an emerging requirement for these applications.

Foreign Object Identification
Machine vision systems are vital in foreign object identification in agriculture, offering a reliable and efficient method for detecting and removing contaminants or foreign materials from agricultural products. These systems utilize high-resolution cameras and advanced imaging algorithms to inspect crops, fruits, vegetables, or processed food items for the presence of foreign objects such as stones, metal fragments, glass shards, or plastic pieces. As the agricultural products move along conveyors or sorting platforms, machine vision cameras capture detailed images of each item, analyzing their visual characteristics and identifying anomalies that deviate from normal product features. By comparing the captured images to predefined templates or thresholds, machine vision systems can accurately detect foreign objects and trigger rejection mechanisms to remove contaminated items from the production line. This proactive approach not only ensures food safety and compliance with quality standards but also minimizes the risk of product recalls and protects consumer health. Additionally, with the integration of machine learning algorithms, machine vision systems can continuously improve their detection capabilities by learning from new data and adapting to evolving types of contaminants, thereby enhancing overall inspection accuracy and efficiency in agricultural processing operations.
Models We Recommend